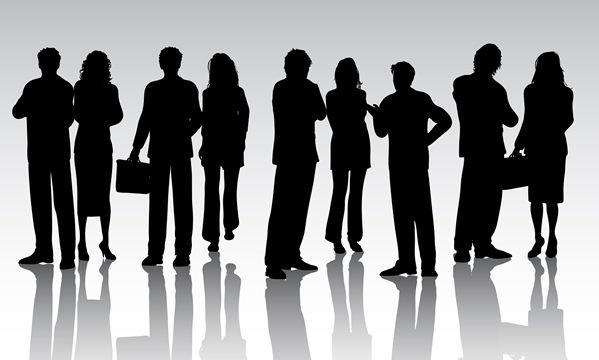
Note: I’ve updated this post as of August, 2015 with even more inclusive and effective diversity searches for LinkedIn.
When it comes to diversity sourcing and recruiting, the first thing that comes to mind for many people is posting jobs on diversity sites and in diverse groups. However, as I have written about many times, posting jobs is an intrinsically limited talent acquisition strategy and it fails to expose you to the “deep end” of the talent pool.
At best, posting jobs can only give you access to approximately 30% of the total talent pool – those active and casual job seekers who will actually take the time to run a search for jobs and apply to an opening.
How can you access the other 70%?
Proactive sourcing, of course!
I’ve spoken at a few conferences this year (HCI, LinkedIn Talent Connect, SourceCon) in which I’ve detailed some Boolean search strings for diversity sourcing on LinkedIn, and I’ve had several requests for the specific searches I’ve demonstrated.
While the search strings I’ve used in my presentations are already posted on the conference websites, I thought it would be a good idea to create and release some new and improved diversity sourcing search strings here for quick and easy access to some “starter” queries.
However, it’s important to know that what I’m publishing is the tip of the iceberg. I have no idea what your particular diversity sourcing need might be, or even what country you’re sourcing in – it’s up to you to adapt what you see here to your specific needs.
While I know some folks will be happy to simply snag the strings, what I really want my readers to get from this post is an understanding of and appreciation for the critical underlying thought process necessary for any successful sourcing endeavor, let alone diversity sourcing.
When it comes to information retrieval, if you can conceive it, you can almost always achieve it – including diversity sourcing – and there are often many different ways to achieving your search goals.
The “magic” of search strings does not lie in the Boolean logic or site specific search syntax, nor does it exist in the keywords and phrases you search for – the true power of search lies within your own mind.
What is Your Diversity Need?
So let’s get back to basics for just a second.
When you’re creating and executing Boolean search strings for talent discovery, you’re really performing information retrieval.
Information retrieval is the activity of obtaining information resources relevant to an information need.
An information retrieval process begins when you enter a query into an information system (e.g., databases, the Internet, social networks, etc.), and queries are simply formal statements of information needs.
So when it comes to diversity sourcing, what’s your information need?
This seems like such a simple question, but I honestly don’t think many people begin their sourcing efforts with this in mind.
Gender Diversity: Women
When it comes to gender diversity recruiting and sourcing, most people tend to think of searching for women’s groups, sororities, women-only sports, and women’s colleges, including searching explicitly for the words “Women,” “Women’s,” and “female” for an exploratory search into all of the various women’s groups.
However, many of these approaches are extremely narrow in scope and low in quantity of results (e.g. “Society of Women” produces a little over 60,000 results in the U.S., and “Association of Female _____” returns just shy of 4,000 results). If you try and search for (“women OR women’s”), while you get nearly 2M results in the U.S., if you scroll through the pages, you can see that there are a fair amount of profiles of men that are returned, and that’s to be expected given that the search terms aren’t exclusive to women’s profiles – they can show up on men’s profiles too.
As an example of something that is less obvious, outside of the box, and more exclusive to women’s profiles would be something I’ve hypothesized, tested, and confirmed on LinkedIn for years – searching for (her OR she), which returns nearly 900,000 profiles.
For those who haven’t seen me present on that search before – do you know why it returns LinkedIn profiles of women?
It works because “her” and “she” can be mentioned in the summary and recommendation sections of women’s profiles.
You would not likely find many LinkedIn profiles of men mentioning “her ” or “she,” although they do exist. (sourcing challenge – do you know how to find them exclusively?)
Yes, (her OR she) is a bit clever, and yes, I’m a bit proud of the discovery, but it clearly demonstrates the fact that all anyone needs to do is *think* about what terms that could be searched for that would be relatively unique to the people you are trying to find and test any ideas you come up with to verify.
While (her OR she) “works” in that it returns predominantly women-only results, it returns less than 1M profiles in the U.S. – so certainly not a big slice of all of the women on LinkedIn.
How could we do better?
Let’s try another more traditional search approach – women’s universities and colleges.
(“Agnes Scott College” OR “Alverno College” OR “Barnard College” OR “Bay Path College” OR “Bennett College” OR “Brenau University” OR “Brescia University College” OR “Bryn Mawr College” OR “Carlow College” OR “Cedar Crest College” OR “Chatham University” OR “College of New Rochelle, The” OR “College of Saint Benedict” OR “College of Saint Elizabeth” OR “College of Saint Mary” OR “Columbia College” OR “Converse College” OR “Cottey College” OR “Douglass Residential College of Rutgers University” OR “Hollins University” OR “Judson College” OR “Mary Baldwin College” OR “Meredith College” OR “Midway College” OR “Mills College” OR “Moore College of Art & Design” OR “Mount Holyoke College” OR “Mount Mary College” OR “Mount St. Mary’s College” OR “Notre Dame of Maryland University” OR “Pine Manor College” OR “Russell Sage College” OR “St. Catherine University” OR “Saint Joseph College” OR “Saint Mary-of-the-Woods College” OR “Saint Mary’s College” OR “Salem College” OR “Scripps College” OR “Simmons College” OR “Smith College” OR “Spelman College” OR “Stephens College” OR “Sweet Briar College” OR “Trinity Washington University” OR “Wellesley College” OR “Wesleyan College” OR “Wilson College” OR “Women’s College”)
That search returns just over 410K results in the U.S.
That’s less than my (her OR she) search, although of course you could use the -/NOT operator to make each search mutually exclusive and to eliminate overlap.
Let’s try a sorority search:
(“Alpha Chi Omega” OR “Alpha Delta Chi” OR “Alpha Delta Pi” OR “Alpha Epsilon Omega” OR “Alpha Epsilon Phi” OR “Alpha Gamma Delta” OR “Alpha Kappa Alpha” OR “alpha Kappa Delta Phi” OR “Alpha Phi Gamma” OR “Alpha Phi” OR “Alpha Pi Omega” OR “Alpha Pi Sigma” OR “Alpha Rho Lambda” OR “Alpha Sigma Alpha” OR “Alpha Sigma Kappa” OR “Alpha Sigma Omega” OR “Alpha Sigma Rho” OR “Alpha Sigma Tau” OR “Alpha Xi Delta” OR “Ceres” OR “Chi Omega” OR “Chi Upsilon Sigma” OR “Delta Chi Lambda” OR “Delta Delta Delta” OR “Delta Gamma” OR “Delta Gamma Pi” OR “Delta Kappa Delta” OR “Delta Phi Epsilon” OR “Delta Phi Lambda” OR “Delta Phi Mu” OR “Delta Phi Omega” OR “Delta Psi Delta” OR “Delta Sigma Chi” OR “Delta Sigma Theta” OR “Delta Tau Lambda” OR “Delta Xi Nu” OR “Delta Xi Phi” OR “Delta Zeta” OR “Gamma Alpha Omega” OR “Gamma Eta” OR “Gamma Phi Beta” OR “Gamma Phi Omega” OR “Gamma Rho Lambda” OR “Gamma Sigma Sigma” OR “Kappa Alpha Theta” OR “Kappa Beta Gamma” OR “Kappa Delta Chi” OR “Kappa Delta Phi” OR “Kappa Delta” OR “Kappa Kappa Gamma” OR “Kappa Phi Gamma” OR “Kappa Phi Lambda” OR “Kappa Phi Zeta” OR “Lambda Pi Chi” OR “Lambda Pi Upsilon” OR “Lambda Psi Delta” OR “Lambda Tau Omega” OR “Lambda Theta Alpha” OR “Lambda Theta Nu” OR “Mu Sigma Upsilon” OR “Omega Phi Beta” OR “Omega Phi Chi” OR “Phi Beta Chi” OR “Phi Mu” OR “Phi Sigma Rho” OR “Phi Sigma Sigma” OR “Pi Beta Phi” OR “Pi Lambda Chi” OR “Sigma Alpha Epsilon Pi” OR “Sigma Alpha Iota” OR “Sigma Delta Tau” OR “Sigma Gamma Rho” OR “Sigma Iota Alpha” OR “Sigma Kappa” OR “Sigma Lambda Alpha” OR “Sigma Lambda Gamma” OR “Sigma Lambda Upsilon” OR “Sigma Omega Nu” OR “Sigma Omega Phi” OR “Sigma Omicron Pi” OR “Sigma Phi Kappa” OR “Sigma Phi Omega” OR “Sigma Pi Alpha” OR “Sigma Psi Zeta” OR “Sigma Sigma Rho” OR “Sigma Sigma Sigma” OR “Tau Theta Pi” OR “Theta Nu Xi” OR “Theta Phi Alpha” OR “Zeta Phi Beta” OR “Zeta Tau Alpha”)
That search returns nearly 1.2M results in the U.S.

Not bad – now we’re over 1,000,000 profiles, which is actually much higher than simply searching for the term “sorority.”
However, instead of trying these more traditional search ideas, let’s try to think of the single most inclusive way of finding women on LinkedIn.
Have any ideas?
Well, what’s more inclusive than first names?
Of course you can search by groups, sororities, and sports, but you can find a larger portion of people by searching by first name.
You might be asking, “How can I possibly create and run a search by all of the female names – there must be thousands?!?!”
Yes, there are thousands, and no, we can’t practically search for all of them – and certainly not in a single search.
However, what you can do is go to a number of websites and find the most popular female names and search for those, which will statistically yield a significant portion of the women represented on LinkedIn. In the U.S., we can use the Social Security Administration website, which conveniently lets you search for the top 200 most common first names for girls and boys by decade.
Fortunately, I’ve done the heavy lifting for you. I copied the top 200 female first names from the 1950’s, 1960’s, 1970’s, 1980’s and 1990’s into Excel, sorted them alphabetically, then removed the duplicates to come up with the most popular 417 names from those 5 decades, which nearly covers the entire span of LinkedIn’s strongest representation.
Then I used those names to create a Boolean OR statement, which looks like this:
(Abigail OR Adriana OR Adrienne OR Aimee OR Alejandra OR Alexa OR Alexandra OR Alexandria OR Alexis OR Alice OR Alicia OR Alisha OR Alison OR Allison OR Alyssa OR Amanda OR Amber OR Amy OR Ana OR Andrea OR Angel OR Angela OR Angelica OR Angie OR Anita OR Ann OR Anna OR Anne OR Annette OR Annie OR April OR Ariana OR Ariel OR Arlene OR Ashlee OR Ashley OR Audrey OR Autumn OR Bailey OR Barbara OR Becky OR Belinda OR Beth OR Bethany OR Betty OR Beverly OR Bianca OR Bonnie OR Brandi OR Brandy OR Breanna OR Brenda OR Briana OR Brianna OR Bridget OR Brittany OR Brittney OR Brooke OR Caitlin OR Caitlyn OR Candace OR Candice OR Carla OR Carly OR Carmen OR Carol OR Carole OR Caroline OR Carolyn OR Carrie OR Casey OR Cassandra OR Cassidy OR Cassie OR Catherine OR Cathy OR Charlene OR Charlotte OR Chelsea OR Chelsey OR Cheryl OR Cheyenne OR Chloe OR Christie OR Christina OR Christine OR Christy OR Cindy OR Claire OR Claudia OR Colleen OR Connie OR Constance OR Courtney OR Cristina OR Crystal OR Cynthia OR Daisy OR Dana OR Danielle OR Darlene OR Dawn OR Deanna OR Debbie OR Deborah OR Debra OR Delores OR Denise OR Desiree OR Destiny OR Diamond OR Diana OR Diane OR Dianne OR Dolores OR Dominique OR Donna OR Doreen OR Doris OR Dorothy OR Ebony OR Eileen OR Elaine OR Elizabeth OR Ellen OR Emily OR Emma OR Erica OR Erika OR Erin OR Eva OR Evelyn OR Faith OR Felicia OR Frances OR Gabriela OR Gabriella OR Gabrielle OR Gail OR Gayle OR Geraldine OR Gina OR Glenda OR Gloria OR Grace OR Gwendolyn OR Hailey OR Haley OR Hannah OR Hayley OR Heather OR Heidi OR Helen OR Holly OR Irene OR Isabel OR Isabella OR Jackie OR Jaclyn OR Jacqueline OR Jade OR Jaime OR Jamie OR Jan OR Jane OR Janet OR Janice OR Janis OR Jasmin OR Jasmine OR Jean OR Jeanette OR Jeanne OR Jenna OR Jennifer OR Jenny OR Jessica OR Jill OR Jillian OR Jo OR Joan OR Joann OR Joanna OR Joanne OR Jocelyn OR Jodi OR Jody OR Jordan OR Josephine OR Joy OR Joyce OR Juanita OR Judith OR Judy OR Julia OR Julie OR June OR Kaitlin OR Kaitlyn OR Kara OR Karen OR Kari OR Karina OR Karla OR Katelyn OR Katherine OR Kathleen OR Kathryn OR Kathy OR Katie OR Katrina OR Kay OR Kayla OR Kaylee OR Kelli OR Kellie OR Kelly OR Kelsey OR Kendra OR Kerri OR Kerry OR Kiara OR Kim OR Kimberly OR Kirsten OR Krista OR Kristen OR Kristi OR Kristie OR Kristin OR Kristina OR Kristine OR Kristy OR Krystal OR Kylie OR Lacey OR Latasha OR Latoya OR Laura OR Lauren OR Laurie OR Leah OR Leslie OR Lillian OR Linda OR Lindsay OR Lindsey OR Lisa OR Lois OR Loretta OR Lori OR Lorraine OR Louise OR Lydia OR Lynda OR Lynn OR Lynne OR Mackenzie OR Madeline OR Madison OR Makayla OR Mallory OR Mandy OR Marcia OR Margaret OR Maria OR Mariah OR Marianne OR Marie OR Marilyn OR Marisa OR Marissa OR Marjorie OR Marlene OR Marsha OR Martha OR Mary OR Maureen OR Mckenzie OR Meagan OR Megan OR Meghan OR Melanie OR Melinda OR Melissa OR Melody OR Mercedes OR Meredith OR Mia OR Michaela OR Michele OR Michelle OR Mikayla OR Mildred OR Mindy OR Miranda OR Misty OR Molly OR Monica OR Monique OR Morgan OR Nancy OR Natalie OR Natasha OR Nichole OR Nicole OR Nina OR Norma OR Olivia OR Paige OR Pam OR Pamela OR Patricia OR Patsy OR Patti OR Patty OR Paula OR Peggy OR Penny OR Phyllis OR Priscilla OR Rachael OR Rachel OR Raven OR Rebecca OR Rebekah OR Regina OR Renee OR Rhonda OR Rita OR Roberta OR Robin OR Robyn OR Rosa OR Rose OR Rosemary OR Roxanne OR Ruby OR Ruth OR Sabrina OR Sally OR Samantha OR Sandra OR Sandy OR Sara OR Sarah OR Savannah OR Selena OR Shannon OR Shari OR Sharon OR Shawna OR Sheena OR Sheila OR Shelby OR Shelia OR Shelley OR Shelly OR Sheri OR Sherri OR Sherry OR Sheryl OR Shirley OR Sierra OR Sonia OR Sonya OR Sophia OR Stacey OR Stacie OR Stacy OR Stefanie OR Stephanie OR Sue OR Summer OR Susan OR Suzanne OR Sydney OR Sylvia OR Tabitha OR Tamara OR Tami OR Tammie OR Tammy OR Tanya OR Tara OR Tasha OR Taylor OR Teresa OR Terri OR Terry OR Theresa OR Tiffany OR Tina OR Toni OR Tonya OR Tracey OR Traci OR Tracie OR Tracy OR Tricia OR Valerie OR Vanessa OR Veronica OR Vicki OR Vickie OR Vicky OR Victoria OR Virginia OR Vivian OR Wanda OR Wendy OR Whitney OR Yesenia OR Yolanda OR Yvette OR Yvonne OR Zoe)
You can then take that Boolean OR statement and enter it into the first name field in LinkedIn. Unfortunately, while that search *used* to work with a free LinkedIn account, that no longer seems to be the case, as I keep getting errors.
It does appear that you can search for about half of that list at once in LinkedIn for free. Try it for yourself here, but I have to warn you, LinkedIn still appears to choke on the search when you try it with a free account. If you can get it to work, you can only view 100 results with a free account, and even then, you will likely run into LinkedIn’s commercial use limit.
The best approach would be to use a premium LinkedIn account in which you can actually fit the entire search into the first name field and view up to 1,000 results at a time.
In LinkedIn Recruiter, my search of 417 female first names returns over 38M results in the U.S. alone.

When it comes to finding women on LinkedIn, how big of a slice does a little over 38M represent in relation to all of the U.S. women on LinkedIn?
Let’s do a little math.
LinkedIn claims about 115M U.S. profiles.
Assuming that this Forbes article is accurate in reporting that LinkedIn has an even ratio of men and women (51%/49%), then there should be approximately 56.4 M female profiles on LinkedIn.
So the ~38.4M results from my search of the 417 first names I ran above could be capturing up to 68% of all of the U.S. women on LinkedIn (38.4M / 56.4M).
Not bad for a single search, and massively more inclusive of any other way of searching for women on LinkedIn (groups, colleges, sororities, etc.)!
If you’re looking for ways to specifically source and recruit women in engineering, I highly recommend you read this LinkedIn post on the topic – it is 1,000 times more effective than trying to hop on the #ILookLikeAnEngineer bandwagon. :)
Of course, if your diversity need is to find male candidates, you can do the exact same thing as above, using the most common male names.
LinkedIn Diversity Sourcing: Racial and Ethnic Diversity
Is your information need to find racially or ethnically diverse candidates?
Well then, all you have to do is think about what might show up predominantly, and ideally only on profiles of people representing specific racial and ethnic groups.
What comes to mind for many people includes searching for groups, fraternities and sororities, and historically black colleges and universities.
Speaking of which, here is a search for 105 HCBU’s:
(“Alabama A&M University” OR “Alabama State University” OR “Albany State University” OR “Alcorn State University” OR “Allen University” OR “University of Arkansas at Pine Bluff” OR “Arkansas Baptist College” OR “Barber-Scotia College” OR “Benedict College” OR “Bennett College” OR “Bethune-Cookman University” OR “Bishop State Community College” OR “Bluefield State College” OR “Bowie State University” OR “Central State University” OR “Cheyney University of Pennsylvania” OR “Claflin University” OR “Clark Atlanta University” OR “Clinton Junior College” OR “Coahoma Community College” OR “Concordia College, Selma” OR “Coppin State University” OR “Delaware State University” OR “Denmark Technical College” OR “Dillard University” OR “University of the District of Columbia” OR “Edward Waters College” OR “Elizabeth City State University” OR “Fayetteville State University” OR “Fisk University” OR “Florida A&M University” OR “Florida Memorial University” OR “Fort Valley State University” OR “Gadsden State Community College” OR “Grambling State University” OR “Hampton University” OR “Harris-Stowe State University” OR “Hinds Community College at Utica” OR “Howard University” OR “Huston-Tillotson University” OR “Interdenominational Theological Center” OR “J. F. Drake State Technical College” OR “Jackson State University” OR “Jarvis Christian College” OR “Johnson C. Smith University” OR “Kentucky State University” OR “Knoxville College” OR “Lane College” OR “Langston University” OR “Lawson State Community College” OR “LeMoyne-Owen College” OR “Lewis College of Business” OR “Lincoln University” OR “Lincoln University of Missouri” OR “Livingstone College” OR “University of Maryland Eastern Shore” OR “Meharry Medical College” OR “Miles College” OR “Mississippi Valley State University” OR “Morehouse College” OR “Morehouse School of Medicine” OR “Morgan State University” OR “Morris Brown College” OR “Morris College” OR “Norfolk State University” OR “North Carolina A&T State University” OR “North Carolina Central University” OR “Oakwood University” OR “Paine College” OR “Paul Quinn College” OR “Philander Smith College” OR “Prairie View A&M University” OR “Rust College” OR “Saint Paul’s College” OR “Savannah State University” OR “Selma University” OR “Shaw University” OR “Shorter College” OR “Shelton State Community College” OR “South Carolina State University” OR “Southern University at New Orleans” OR “Southern University at Shreveport” OR “Southern University and A&M College” OR “Southwestern Christian College” OR “Spelman College” OR “St. Augustine’s College” OR “St. Philip’s College” OR “Stillman College” OR “Talladega College” OR “Tennessee State University” OR “Texas College” OR “Texas Southern University” OR “Tougaloo College” OR “Trenholm State Technical College” OR “Tuskegee University” OR “University of the Virgin Islands” OR “Virginia State University” OR “Virginia Union University” OR “Virginia University of Lynchburg” OR “Voorhees College” OR “West Virginia State University” OR “Wilberforce University” OR “Wiley College” OR “Winston-Salem State University” OR “Xavier University of Louisiana”)
While people have been leveraging HCBU’s for years in their diversity sourcing efforts, unlike most (all?) ATS’s, resume databases, and Internet search engines, LinkedIn is the only place that I am aware of that can handle 3,000+ character Boolean search strings to allow you to search for all of them at once (thank you LinkedIn!).
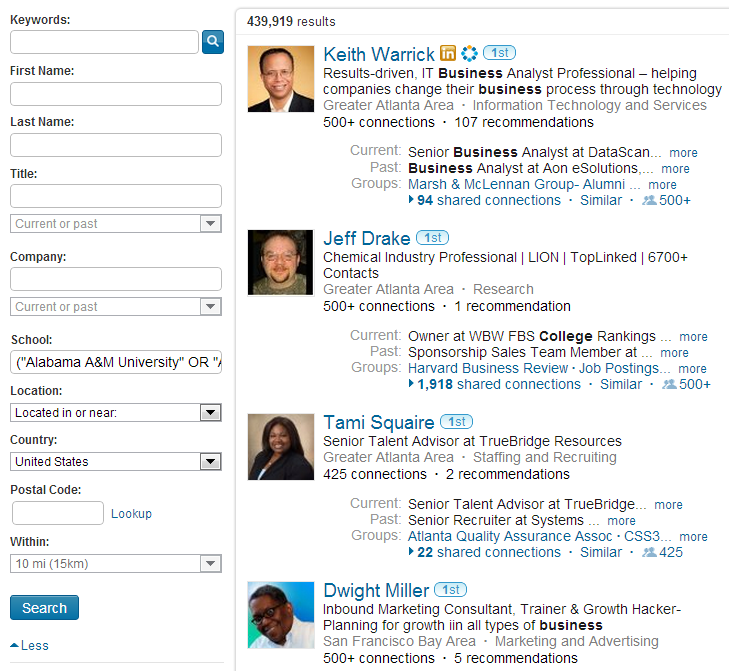
While anyone who performs diversity sourcing in the U.S. is familiar with HCBU’s, not everyone knows that you can also search for colleges and universities that have a high percentage of other racial or ethnic groups, such as historically Native American colleges and universities – here’s the search on LinkedIn – it all comes down to your specific need.
If you’d like to take the fraternity/sorority approach, here is a LinkedIn search for African American fraternities and sororities.
(“Sigma Pi Phi” OR “Alpha Phi Alpha” OR “Kappa Alpha Psi” OR “Omega Psi Phi” OR “Phi Beta Sigma” OR “Sigma Rhomeo” OR “Wine Psi Phi” OR “Iota Phi Theta” OR “Phi Delta Psi” OR “Delta Psi Chi” OR “Beta Phi Pi” OR “MALIK Fraternity” OR “Sigma Phi Rho” OR “Phi Rho Eta” OR “Gamma Psi Beta” OR “Alpha Kappa Alpha” OR “Delta Sigma Theta” OR “Zeta Phi Beta” OR “Sigma Gamma Rho” OR “Phi Delta Kappa” OR “Iota Phi Lambda” OR “Eta Phi Beta” OR “Gamma Phi Delta”)
Depending on need, you can also construct queries for Asian American, Latino, LGBT, and other cultural interest fraternities and sororities.
As with all information retrieval efforts, it comes down to your specific information need and discovering ways of achieving those needs.
LinkedIn Diversity Sourcing: Surname Search
Speaking of specific diversity sourcing needs, you may be able to experiment with searching for last names to achieve your diversity sourcing goals.
Just as a quick and random example, here is search for the top 100 Chinese surnames:
(Lǐ OR Wáng OR Zhāng OR Liú OR Chén OR Yáng OR Zhào OR Huáng OR Zhōu OR Wú OR Xú OR Sūn OR Hú OR Zhū OR Gāo OR Lín OR Hé OR Guō OR Mǎ OR Luó OR Liáng OR Sòng OR Zhèng OR Xiè OR Hán OR Táng OR Féng OR Yú OR Dǒng OR Xiāo OR Chéng OR Cáo OR Yuán OR Dèng OR Xǔ OR Fù OR Shěn OR Zēng OR Péng OR Lǚ OR Sū OR Lú OR Jiǎng OR Cài OR Jiǎ OR Dīng OR Wèi OR Xuē OR Yè OR Yán OR Yú OR Pān OR Dù OR Dài OR Xià OR Zhōng OR Wāng OR Tián OR Rén OR Jiāng OR Fàn OR Fāng OR Shí OR Yáo OR Tán OR Shèng OR Zōu OR Xióng OR Jīn OR Lù OR Hǎo OR Kǒng OR Bái OR Cuī OR Kāng OR Máo OR Qiū OR Qín OR Jiāng OR Shǐ OR Gù OR Hóu OR Shào OR Mèng OR Lóng OR Wàn OR Duàn OR Zhāng OR Qián OR Tāng OR Yǐn OR Lí OR Yì OR Cháng OR Wǔ OR Qiáo OR Hè OR Lài OR Gōng OR Wén)
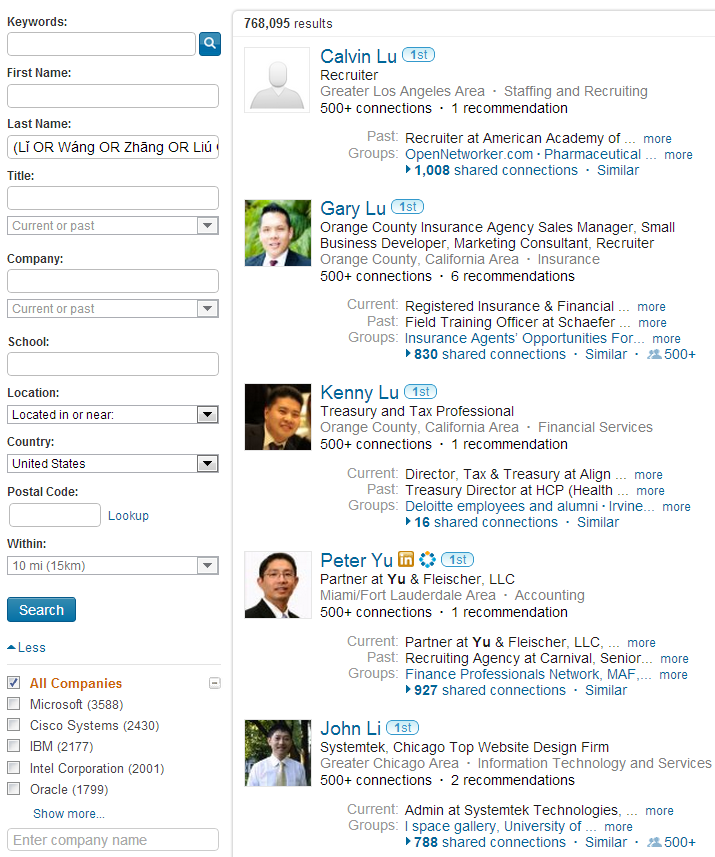
You could of course combine this approach with one or more of the gender diversity Boolean search strings if that would help you achieve your diversity sourcing goals.
If you’re wondering if anyone actually performs these kinds of diversity-focused sourcing strategies, the answer is a resounding “yes!” I have people approach me all the time at conferences referencing how they’ve successfully leveraged the diversity sourcing strategies and tactics outlines in this post. Recently, while attending and speaking at the always awesome Talent42 technical recruiting conference in Seattle, I had someone tell me how they leveraged the most common Korean surnames to find a bilingual engineer which made short work of the otherwise seemingly impossible challenge.
Final Thoughts on LinkedIn Diversity Sourcing
To be sure, what I’ve demonstrated here has some obvious limitations and is far from perfect, but it does effectively illustrate that you do have some creative proactive sourcing options for underrepresented gender and racial/ethnic groups in your organization, allowing you to move beyond relying solely on posting jobs and hoping to get qualified (and diverse!) applicants from active candidates.
Proactive diversity sourcing has the distinct benefit of giving you access to the deeper end of the talent pool – those people (typically more than 2/3rds of any given population) who aren’t actively seeking employment and thus cannot be reached through any form of job advertisement, no matter where you post or share it.
Ultimately, what I really wanted to accomplish by writing this article was to get you thinking a little bit differently when it comes to diversity sourcing and sourcing in general. Effective sourcing, diversity or otherwise, isn’t about Boolean search strings – it’s about critical thinking and always seeking to step outside of the box to find ways to meet your information needs.
As an added bonus for reading this entire post, here is a LinkedIn search of the top 200 most popular female first names in the United States from the 1950’s, 60’s, 70’s, and 80’s de-duped to the most popular 354 names from those decades, which captures the 23-62 year old demographic, which nearly covers the entire span of LinkedIn’s strongest representation.
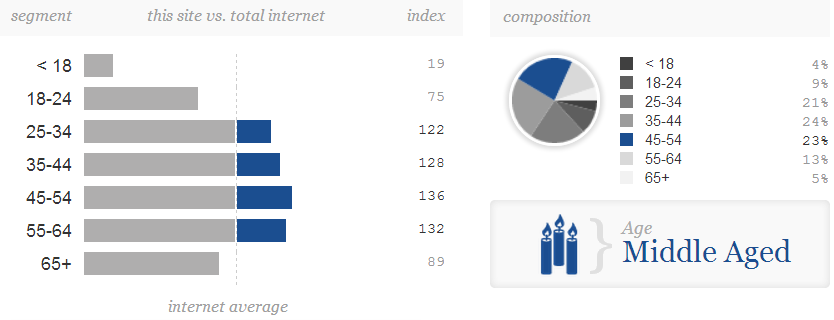